

First, on the kind of annotation scale employed, i.e., either discrete or continuous. Similarly, the approaches to step (ii) vary along the following two main aspects.
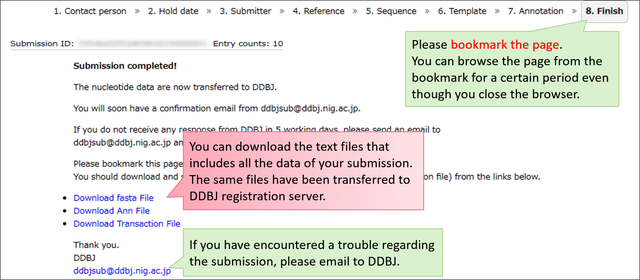
For example, during step (i) different modalities like physiological signals 5, 7, 8, speech 9 and facial-expressions 10, 11 can be acquired. For undertaking steps (i) and (ii) several different strategies are used. To overcome this limitation, the standard AC processing pipeline 6 involves: (i) acquiring measurable indicators of human emotions, (ii) acquiring subjective annotations of internal emotions, and (iii) modelling the relation between these indicators and annotations to make predictions about the emotional state of the user. Addressing this shortcoming is the aim of the interdisciplinary field of Affective Computing (AC, also known as Emotional AI), that focuses on developing machines capable of recognising, interpreting and adapting to human emotions 3, 4.Ī major hurdle in developing these affective machines is the internal nature of emotions that makes them inaccessible to external systems 5. These advancements in interpreting explicit human intent, while highly commendable, often overlook implicit aspects of human–human interactions and the role emotions play in them. For example, services like customer support and patient care, that were till recently only accessible through human–human interaction, can nowadays be offered through AI-enabled conversational chatbots 1 and robotic daily assistants 2, respectively. The field of Artificial Intelligence (AI) has rapidly advanced in the last decade and is on the cusp of transforming several aspects of our daily existence. The validity of the emotion induction, as exemplified by the annotation and physiological data, is also presented. The dataset consists of the physiological and annotation data from 30 participants, 15 male and 15 female, who watched several validated video-stimuli. In parallel, eight high quality, synchronized physiological recordings (1000 Hz, 16-bit ADC) were obtained from ECG, BVP, EMG (3x), GSR (or EDA), respiration and skin temperature sensors.

For this purpose, a novel, intuitive joystick-based annotation interface was developed, that allowed for simultaneous reporting of valence and arousal, that are instead often annotated independently. The Continuously Annotated Signals of Emotion (CASE) dataset provides a solution as it focusses on real-time continuous annotation of emotions, as experienced by the participants, while watching various videos. As a result, proper emotion assessment remains a problematic issue. Discrete, indirect, post-hoc recordings are therefore the norm. In research, a direct and real-time inspection in realistic settings is not possible. From a computational viewpoint, emotions continue to be intriguingly hard to understand.
